IFS co-organises FAIR Learning Analytics Workshop
- News
- Events
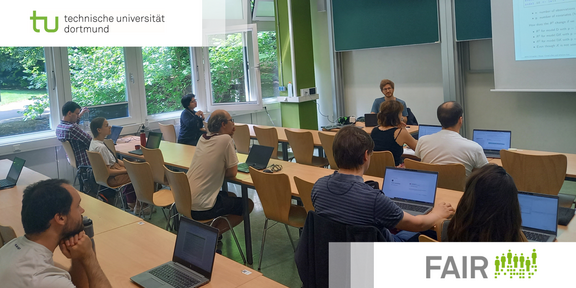
The interdisciplinary research profile FAIR is holding a Learning Analytics Workshop co-organised by the IFS from September 25–27 .
The interdisciplinary research profile FAIR is organizing workshop on learning analytics at the TU Dortmund University from September 25–27. Learning analytics is the collection, analysis, and visualization of detailed data about learning processes, and aims to provide learners with information about their learning process, as well as researchers the possibility to conduct fine-grained analyses on learning processes.
The two-part workshop starts with an introduction to learning analytics. On September 25, JProfessor Ioana Jivet from the University of Hagen and JProfessor Maren Scheffel from the Ruhr University Bochum will provide an overview of the effective design of dashboards and data visualization in the learning context, including possibilities to designing your own dashboards. On September 26, researchers from the University of Mannheim – Dr. Jan Delcker, Joana Heil and Yvonne Hemmler – will give an introduction to data-driven support for learning processes. The second part of the workshop will focus on the participants' current research. In addition to presentations of their own research projects on learning analytics and related fields, the aim is to initiate collaborations between participants of the workshop.
The workshop is organized by researchers from the IFS – Dr. Jakob Schwerter, Dr. Thomas Brüggemann, Dr. Elisabeth Graf – and the chair of statistical methods in social sciences – Professor Philipp Döbler. Interested researchers can register online for the workshop until September 7 or contact the FAIR workshop team at workshop.fairtu-dortmundde.
The interdisciplinary team in the FAIR profile focuses on the development, testing, and application of innovative approaches for dealing with large amounts of data and small numbers of cases in order to optimise prognosis and intervention. The scientists at the IFS are primarily involved in intervention research and analysing large-scale assessments in the education sector, as well as simulation studies on dealing with missing values and the use of tree-based methods for data with missing values.